Digitalization Review for American SMEs
Volume 9, Issue 4, Page No 93-101, 2024
Author’s Name: Dharmender Salian, Steven Brown 2, Raed Sbeit 3
View Affiliations
School of Computer and Information Sciences, University of the Cumberlands, Williamsburg, 40769, USA
a)whom correspondence should be addressed. E-mail: dsalian0302@ucumberlands.edu
Adv. Sci. Technol. Eng. Syst. J. 9(4), 93-101 (2024); DOI: 10.25046/aj090410
Keywords: Industry 5.0, Big Data, Digitalization
Export Citations
SME big data maturity models will be reviewed in this study to identify systematic publications related to the subject. For SMEs to remain competitive, digitalization is essential. Due to limited resources, SMEs need to be more proactive in digitalization. Still, the benefits, such as operational efficiency, cost reduction, quality improvement, and innovative culture, make digitalization attractive and valuable to customers. In recent years, there has been an increase in the use of big data techniques in operations. The paper discusses big data applications in SMEs through the lens of a big data maturity model. This paper met two objectives. First, this paper summarizes the most commonly used maturity models in the existing literature. Second, existing Big Data maturity models have limitations. Moreover, this paper outlines key considerations for selecting a Big Data maturity model to support data-driven decisions. Based on the Big Data maturity dimensions, further work aims to develop a new Big Data maturity model.
Received: 30 April 2024, Revised: 18 July 2024, Accepted: 31 July 2024, Online: 18 August 2024
1. Introduction
This paper is an extension of work initially presented at the IEEE Conference [1]. The heterogeneous data sets in big data contain different types of information. Organizations worldwide have been focusing on big data for the last decade. Real-time decision-making relies on statistics, econometrics, math, simulations, optimizations, and other techniques for collecting and analyzing high-speed data from multiple sources. [2]. Big data must be understood and adapted to specific uses and requirements in most organizations [3]. Big data allows managers to monitor organizational processes, assets, production units, and supply chains [4]. Organizations can identify their competitors with the help of current and new web data [5]. Analyzing big data can also help producers discover what their customers are doing, what they are complaining about, and what they are asking.
Information management and strategic use are increasingly important to organizations and industries [6]. Organizations can gain a competitive edge by collecting big data. Costs and time can add up when acquiring organization-specific data [7]. Many factors go into collecting and storing data, analyzing the data correctly, and even knowing what questions to ask and which data to collect. [7]. Big data requires new skills that traditional analysts still need to gain [6]. The use of Big Data can fundamentally transform businesses through radical disruption, minor incremental improvements, or a complete reimagining of the business model. Using big data’s volume, variety, and velocity can lead to new insights and better decision-making [8]. Digital technology generates much data from various sources, such as manufacturing, businesses, education, and entertainment. [9]. Due to its popularity, many fields have incorporated machine learning, cognitive science, and the semantic web [9]. Data security, privacy, and ethical concerns hinder big data’s potential.
Strategic planning and execution guide business decisions [10]. Strategic planning aims to get things done, so intelligence needs to be deployed [10]. A company’s strategy must be positioned strategically with the right vision as online, social media, and big data continue to grow in importance [11]. Big data can help businesses grow internationally and improve their performance in critical areas like international market orientation [11].
In addition to managing inventory, controlling quality, and minimizing costs, hybrid technology systems assist companies with their operations. Technology can be valued by core use cases instead of expert systems, robot localization, and visual surveillance [8]. There are 61.7 million small and medium businesses (SMEs) in America, which account for 46.4% of the workforce [12]. Governments and large tech companies are funding big data applications in advanced economies. Big data is being reviewed in engineering and manufacturing since previous reviews ignored them.
SMEs need to catch up to larger firms in their adoption of digital technologies, and digital adoption by SMEs is still confined mainly to essential services, with adoption gaps increasing with the sophistication of technologies. SMEs can significantly benefit from digital systems, but their development could be more expensive, time-consuming, and skill-intensive. Since the COVID-19 crisis, SME digitalization has accelerated, but barriers remain.
SMEs comprise most companies and industries in most countries and regions, and their contribution to inclusive and resilient societies is critical. Due to the information technology revolution, SMEs’ identity and position have changed dramatically over the past two decades. Due to this period, they have become more vulnerable to global competition, but they also have acquired competencies and opportunities to reach previously unimagined audiences.SME adoption of digital transformation poses many challenges for governments, including encouraging digital adoption by SMEs, supporting SME training and upskilling, and strengthening managerial skills.
A growing number of small businesses have identified the importance of digital Infrastructure as a significant driver of long-term financial growth and adaptability. To remain competitive in an evolving digital landscape, SMEs must keep these trends in mind. Investing in digitalization can enable SMEs to gain valuable insights from their data, increase efficiency, reduce costs, improve competitiveness, and create more significant opportunities for scalability and growth. It may involve a substantial upfront cost in the medium to long term. Digitalization presents both opportunities and challenges for SMEs in contemporary business.
Digital Transformation in automotive manufacturing means using digital tools to improve efficiency and reduce costs. Optimizing production planning and improving quality is made possible by data-driven analytics and artificial intelligence. With digital transformation, businesses can reduce production costs and manufacturing time as they face cutthroat competition. Automated robots and automation help companies analyze data in real time, improving productivity, while artificial intelligence, additive manufacturing, and the Internet of Things revolutionize product design. Electric cars are becoming more popular as eco-friendly vehicles increase demand, so automakers need new suppliers. Models of maturity (M.M.s) serve as tools for answering the following questions.
RQ1 In automotive manufacturing, what role does big data maturity play?
RQ2 What are the different stages of maturity described in the literature review?
RQ3 Big data maturity models have what characteristics and goals?
A systematic review of big data M.M.s determines the dimensions to assess. The extensive data maturity assessment can help organizations evaluate their capabilities, identify gaps, and create a roadmap for building more successful big data programs [13]. This study will fill the research gap and guide future studies considering these dimensions. Following is a breakdown of the rest of the paper: Sections 2 and 3 present a SWOT and PESTLE analysis of SMEs. Section 4 is about Big Data applications. Digital Strategy is in section 5. The sixth section discusses big data. Section 7 provides a literature review. Section 8 is about the requirements for a new SME maturity model. Section 8 describes AI MM for the aerospace industry. Section 9 discusses future challenges, while section 10 summarizes the study’s results.
2. SWOT
Table 1: SWOT Analysis of SMEs
Strength | Weakness |
· Adaptable and more flexible organization structure. · Experience, knowledge, accomplishments, and skills. · Constant product innovation · Facilities in developing nation · Electric vehicles increased their market shares. · Incentives and support from the government |
· Bargaining power of consumers · Government regulations · Investments are expensive · R&D facilities are not good enough · Lack of skilled workers · A declining aftermarket |
Opportunities | Threats |
· Aid from the government. · Meeting customer needs and making the organization more efficient. · Production costs are lower. · Batteries and electric cars have led to new supply chains. · Commercial vehicles are becoming more multifunctional. |
· Rising competition · Sluggish economy · Competition in the market. · The inflation rate is high · An economic downturn · Enterprising newcomers |
3. Pestle Analysis
New products and projects are launched in an environment tracked by pestle analysis (see Table 2).
Table 2: SME PESTLE Analysis
Political | Economical |
· U.S. auto sales are growing. | · Automobile industry hit by pandemics. |
Social | Technological |
· The auto industry needs skilled talent. | · The digital revolution is taking off. |
Legal | Environmental |
· The auto industry has regulations and restrictions. | · Manufacturing’s environmental impact. |
4. Big Data Applications in Manufacturing
Intelligent systems have transformed smart manufacturing through the digital revolution. New methods for developing and deploying big data could benefit the manufacturing industry. The vast majority of proposed solutions in manufacturing build a big data platform from scratch by installing and testing each tool individually, owing to the fast-changing nature of many of the tools. As big data and manufacturing knowledge converge, smart manufacturing becomes more actionable in real-time. Big data are collected and analyzed for timely information, but manufacturing industries may only know the best approach with domain expertise. Manufacturers can create actionable knowledge by combining manufacturing knowledge with timely information in big data.
Machines, products, and supply chains are all examples of big data gathered in the manufacturing industry. Big Data Manufacturing finds previously unavailable insights into efficiency, productivity, and quality. With industrial Internet of Things (IIoT) sensors, manufacturing execution systems (MES) (software applications that track and control production processes on the factory floor), and industrial control systems, manufacturers are gathering a lot of big data from different sources throughout their operations. (crucial for managing automated processes in manufacturing). Many production activities affect yield, so manufacturers must diagnose and correct process errors in detail. Analytics provides actionable insights and patterns by analyzing data using statistical models, machine learning, and artificial intelligence. The capabilities of conducting sophisticated statistical analyses are now available to global manufacturers across various industries and geographies. Companies are now aggregating and analyzing previously isolated data sets to uncover valuable insights, which requires long-term planning and investment. By identifying opportunities for new products and improvements in existing processes, data-driven insights can give manufacturers a competitive advantage.
SMEs need more funding and talent. Currently, they are reskilling their employees and adopting a new employment model that emphasizes selecting employees with various skill sets. To understand big data, SMEs must develop extensive data knowledge and education, workplace training, recognition, awareness, and promotion. Everyone must understand the benefits of comprehensive data adoption regardless of level or type of organization.
- Maintenance Regulation
Integration of Machine Learning (ML) and Big Data (B.D.) into IoT (Internet of Things).
- Quality Checks
Digitalization has improved the accuracy and efficiency of manufacturing quality assurance (Q.A.).
- Supply Chain Management
Traditional systems must change to keep up with the complexity of supply chain management (SCM). Analyzing big data requires tools, processing systems, and algorithms to interpret insights. Making better decisions requires better data integration; automation is only the beginning.
- Risk Evaluation
Big data can help organizations predict and calculate risks so they can take preventative measures. When assessing risks, one must consider trends with unique impacts, including human talent, production materials, and global economic developments. Additionally, losses and low margins can pose risks.
- Production Optimization
Data analytics can improve aging manufacturing systems. The use of big data ranges from forecasting to enterprise performance management.
- Sustainable Development
Big data can prevent resource waste. Preventing excessive production costs begins with monitoring supply chain management and machine management.
5. Digital Strategy
Digitization converts analog to digital. As the business model changes, a new revenue and value-producing opportunity arises. In today’s workplace, industry, and region, digital skills are a must. Companies are implementing digital technologies, which means jobs are changing. Whether transforming business processes or shifting work roles, automation is critical to digitalization. Transparency and efficiency boost the organization’s bottom line with digitalization.
As a result of technology, manufacturing companies are becoming more efficient, customer-centric, and competitive. Manufacturers can improve their products with the help of artificial intelligence, machine learning, and big data analytics. Manufacturers can improve their products with the help of artificial intelligence, machine learning, and big data analytics. In addition to building brand equity, increasing employee effectiveness, and increasing profits, industries and businesses must have strategic plans. Figure 1 shows Firm inversion (14).
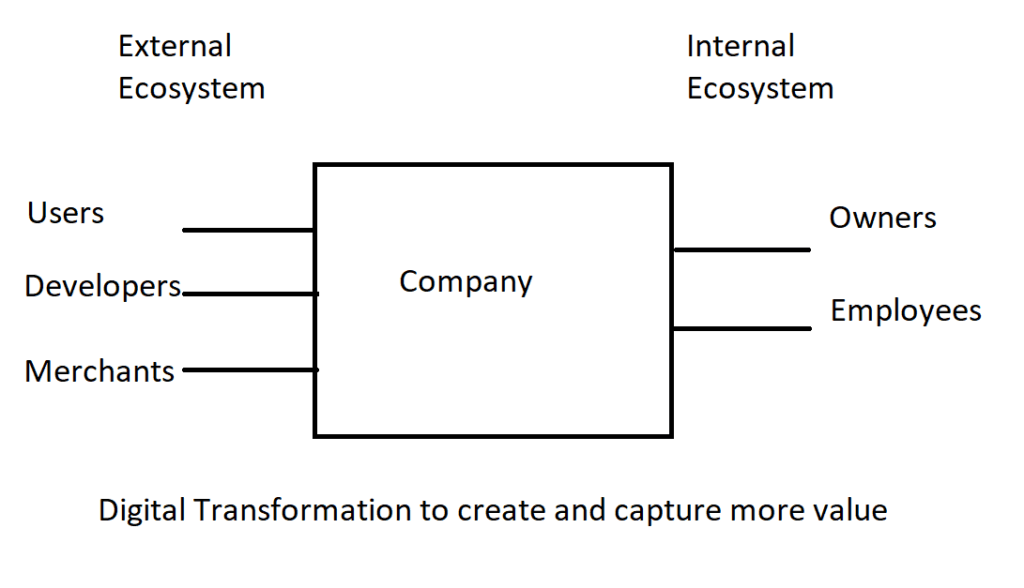
The inversion of a company is where the value creation is due to digital transformation. Creating value on their own is less crucial than orchestrating value with other firms, so users, developers, and merchants need to be able to partner with digital investments at scale to support firm inversion. Inverted firms rely on others’ resources rather than controlling their own, and to enable this, managers need to switch from controlling to enabling. An external ecosystem can thrive if companies coerce partners into sharing ideas, investments, and effort. Leaders are responsible for encouraging and accelerating multiparty collaboration within and outside their organizations.
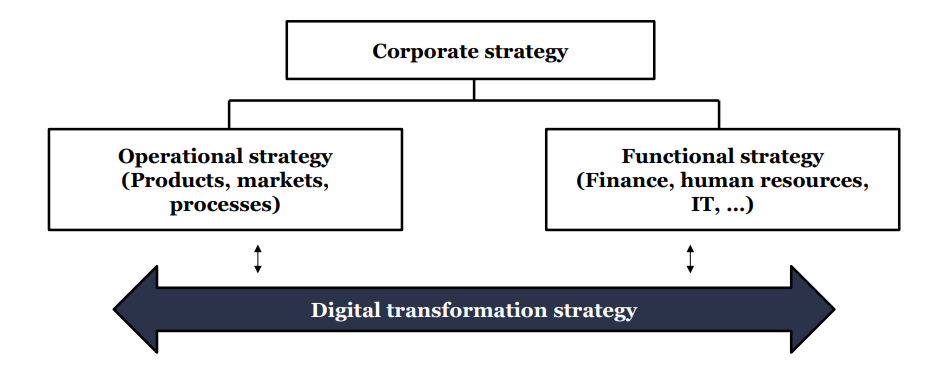
Organizations, products, and processes are all being transformed by digital transformation. Digital transformation should align organizational and functional strategies (see Figure 2). Financials play a role in both driving and constraining transformation. Companies can finance transformations both internally and externally. The key to transforming business is to create new opportunities. Whatever the industry or firm, digital transformation strategies have some things in common: technology, value creation, structure, and finance (see Figure 3).
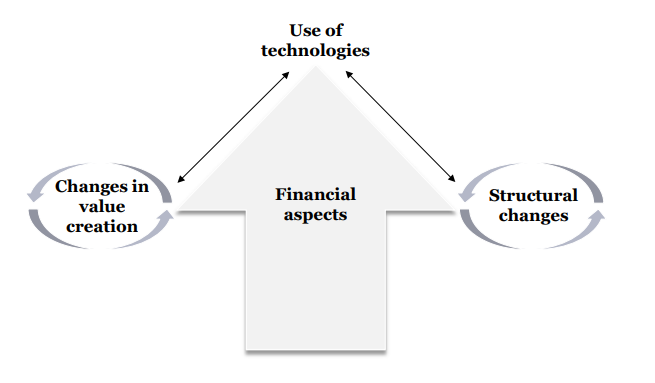
Porter recommends three generic strategies for any business: differentiation, leadership, and focus. In the digital transformation, the inversion of a company that makes the most money reshapes how companies create value. Customized transformation measures can help achieve this. Companies can do this when they stop creating value independently and start orchestrating it with each other. Partnering with users, developers, and merchants at scale is the key to success. Network effects are not common in standalone companies, but they might occur if they look at their business frame in an ecosystem context. High market caps do not come from automation or transferring labor to capital but from coordinating external value creation. Shared value can help companies get multiple benefits.
Economies and markets are suffering from Uber’s and Airbnb’s systemic effects. Digital disruption – or radical digital innovation – and its broader systemic effects get much attention. From their perspective, companies heavily invested in old conditions tend to be disrupted by digital disruption. Digital processes and artifacts can radically change operations for established companies. There are a lot of digital innovations that have triggered and spurred the digital revolution, some of which might disrupt traditional industries. Embracing the digital transformation of manufacturing operations is critical for manufacturers seeking unprecedented efficiency and quality control.
6. Big Data and SME
Big data for businesses, the I.T. industry, and researchers are associated with challenges and opportunities. These days, SMEs need more resources to deal with big data. The high speed and volume of data exchange in industries have resulted in big data becoming one of the topics of discussion among managers and decision-makers these days because it provides the information needed to pinpoint inefficiencies in their operations and, as a result, resolve these issues. SMEs are more flexible and adaptable to technology and change than large firms, and managers of SMEs might be influenced by significant characteristics such as competition pressure, financial resources, and talent shortages when making decisions regarding extensive data adoption. This study’s findings reinforce that technology and innovation are priorities for the growth of SMEs, and big data will contribute significantly to this growth. Using big data in SMEs gives organizations greater flexibility, efficiency, responsiveness, and ability to anticipate and meet customer needs
SMEs benefit from big data by offering real-time solutions to issues in every industry and fostering alliances. Openness helps to make decisions, and SMEs are chosen explicitly within the context. Because of their overall position in the economy, SMEs have the advantage and flexibility to adjust to changes faster toward efficiency, and a slight change can have a significant macro-level impact. Nevertheless, there are several contentious issues associated with Big Data, including storage, processing, and generating useful information from it, as well as privacy and security concerns. With the addition of machine-collected data (e.g., intelligent counters and sensors), the datasets can grow to enormous sizes, bringing about the possibility of improved decision-making and performance outcomes. The fourth industrial revolution and global digitization of consumers, customers, and providers have created abundant data that firms must learn to use [15]. Technology such as the Internet of Things (IoT), which has the potential to lead to many developments, is essential when it comes to exploring big data. In addition, manufacturers can use IoT to monitor machinery and track parts in real-time.
Furthermore, IoT connects everything. The internet allows us to connect things in our everyday lives, like cars, T.V.s, washing machines, pumps, shipping containers, and machinery. Cloud-based technology and mobile devices with connections to all these things mean SMEs must be bold in taking business-related risks for advancements.
Transparency makes data available to relevant users as promptly as possible, while open transparency improves data quality, consistency, reliability, availability, and accessibility. As a result, open, transparent data is valuable for improving product offerings, reducing time-to-market, and improving engineering processes. Data transparency is achieved by effectively managing people, data, tools, and intent collectively through proper Big Data management, and the most critical aspects of BDM are data governance, business intelligence/data warehouse management, and data quality management. BDM requires knowledge of new Big Data technologies and business practices. Processed and refined data becomes more valuable over time but also loses value over time. To overcome this challenge, BDM should be automated. Automated BDM processes make it easier to manage the data more efficiently and effectively while freeing up human resources simultaneously.
The West’s major players in Big Data are Alphabet (Google), Amazing, Facebook, and Apple. [16]. Technology leadership, research, and the development of new processes can help develop innovative processes. Organizations must still utilize big data and adapt it to specific needs and requirements [17]. Businesses can increase performance and expose variability by creating and storing more digital transactional data. SMEs should focus on the growth opportunities that result from Big Data when evaluating its use and potential. Segmentation of customers can improve products and services, and Big Data can be used to analyze in-depth data for correlations, risks, and opportunities and to do predictive maintenance, demand forecasting, process optimization, inventory planning, and market segmentation, analysis, and forecasting. Researchers have a consensus that Big Data is transforming information sciences, manufacturing, retail, and healthcare [18]. A big data model for SMEs is possible with the leaders’ support, mandate, and trust, and data from across all divisions will only be shared when management has given it a clear direction and a clear picture of the results. Lastly, Big Data can significantly improve revenue, cost, profit, and growth through improved productivity, efficiency, and new business creation.
7. Literature Review
An extensive literature review can help identify knowledge gaps, generate insights, and provide helpful advice. This paper reviews previous studies on big data maturity models and discusses developments in the future using an in-depth literature review.
Most organizations are still experimenting with big data. Big Data strategies and Big Data technology can benefit businesses by increasing value, decreasing costs, improving operational efficiency, and streamlining operations. Automation, employee productivity, and streamlining can all be achieved with big data. Simplified operations and reduced downtime are the results of Big Data.
The maturity model provides layered levels of accomplishment for assessing maturity in these areas and pinpointing areas for improvement for stakeholders to plan. Big data has different maturity models with different names, levels, and content. The following sections will summarize these models.
An organization’s architecture is one way to describe its prominent data structure. The data organization model describes how different systems integrate. An organization’s competitive advantage depends on data quality; thus, a maturity model is critical. [28]. Table 3 shows maturity models. Digital transformation for SMEs is a specialty of others, while some specialize in big data.
Table 3: Comparing big data dimensions overall
Author | [19] | [20] | [21] | [22] | [23] |
Connectivity | X | X | X | X | |
Resilience | X | X | X | ||
Sustainability | X | X | |||
Expansive Growth | X | X | X | ||
Strategy | X | X | X | X | |
Leadership | X | X | X | X | |
Customers | X | X | X | ||
Culture | X | X | |||
Production | X | X | X | X |
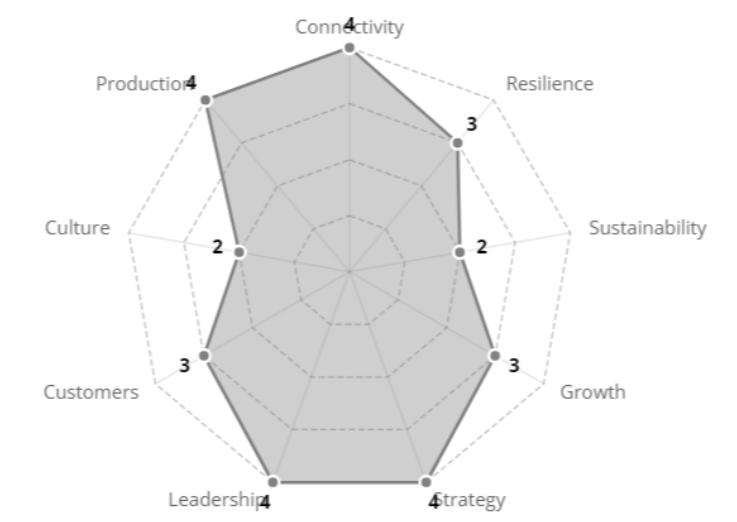
By identifying the shortcomings, the big data maturity model can be improved (see Figure 4). The maturity models discussed above only work for SMEs, are prevalent, and may result in inaccurate results. Regarding big data MM for automotive small and medium enterprises, the maturity assessment dimensions cover only some sizes and capabilities. Below are some critical dimensions of big data assessment, so a new big data MM should cover these dimensions.
8. Requirements for a new SME maturity model
Model construction based on an organization’s future big data needs to identify strengths and areas for improvement and prioritize reaching higher levels of big data maturity. These maturity stages can serve the research gap:
- Nascent: Beginners need strategic focus. Regarding environmental pursuits, organizations are still in the exploratory phase, looking at the benefits of big data.
- Innovation: In the innovation stage, companies use big data techniques to reduce costs and increase revenue. A culture of innovation is being developed, focusing on environmental benefits.
- Integration: During the Integration stage, organizations have relatively straightforward business strategies for using big data, and their processes, Infrastructure, and investments align with what big data requires. Big data-embracing culture and environment policies are in place in subunits pursuing big data innovations.
- Mature: A big data-focused work culture is characteristic of organizations at the mature stage of big data maturity. The organization employs big data-enabled action plans to exhibit competitive superiority. The matrix below compares the four maturity stages with the selected big data dimensions.
Table 4: Most influencing parameters for each maturity stage
STAGE | ||||||
Parameter | Nascent | Innovation | Integration | Mature | ||
D I M E N S I O N |
Leadership | Evaluate Objectives | Organizational priorities | Expectations and concerns | Integration planning | driving functional excellence |
Measure confidence | ||||||
Expansive growth | Successful transformation | Setting clear, attainable goals | A collaborative and adaptive growth process | Identifying areas of concern and achieving integration goals. | Attained a stable and efficient operational state | |
Grow healthily | ||||||
Strategy | Governance | The goal is undefined, and no budget allocation | The focus is on cost leadership and efficiency | Aligned with the overall business strategy | Business development and competitiveness | |
Cost leadership | ||||||
security | ||||||
Resiliency | Handle adversity | Risk initiation and command structure in place | The resilience of the market for essential supplies | System and Infrastructure established. | Professional and responsible. An insight into the market. | |
insecurity | ||||||
Sustainability | Sustainability business practices | Taking advantage of opportunities | Exploring benefits | Participating in stakeholder engagement | Environmentally friendly and have profit | |
Culture | Culture shift | Getting the most out of big data | A culture that embraces big data | Embracing big data and evolving culturally | A proactive mindset and innovative decision-making | |
Innovation mindset | ||||||
Customer | Engaging customers | Engaging customers in a more meaningful way | Establishing a customer-centric approach | Customer-centricity and clearly defined accountability | Fulfill customer desires and needs | |
Production | Empower employees | Developing but not prioritized | Big data professionals in charge need more enterprise-level centralization despite their expertise. | Decisions align with the larger ecosystem and boardroom decisions. | Automate and reduce inefficiencies |
9. Big Data MM for the Automotive SME Market
Data maturity is the ability of an organization to use its data effectively. Companies can develop best practices for managing big data by assessing their data maturity. The maturity model assesses an organization’s readiness, identifies its weaknesses, and identifies its capabilities. It is vital to fix those weaknesses and improve the assessment to advance. Organizations should assess their extensive data capabilities before moving on to maturity models since they need more standardized development methodologies. The goals of big data maturity models are:
- Analyze big data in critical areas of the organization with this tool.
- We are setting milestones during development.
Data maturity leads to increased revenue and lower costs. As part of Industry 5.0, big data maturity models should support sustainability, resilience, and human-centered design (see Figure 5). Productivity and efficiency are only part of the Industrial 5.0 vision [24]. Worker well-being is prioritized and emphasizes the industry’s role in society. Instead of just assessing technology, industry 5.0 focuses on value rather than technology. Industrial output and profit alone are no longer enough to account for environmental and societal costs. Investing in automation, digitization, and artificial intelligence is essential, but not at the expense of sustainability and human beings. People and societies should benefit from technology, which is employed to make this ideal a reality. A sustainable approach to natural resources relies on repurposing, recycling, and reuse. Thus, it reduces waste and the negative impacts on the environment. Green solutions will be vital for companies (and society as a whole) in the next few decades. A resilient supply chain and production means making them more resilient to interruptions and ensuring that critical Infrastructure is available and supported during crises.
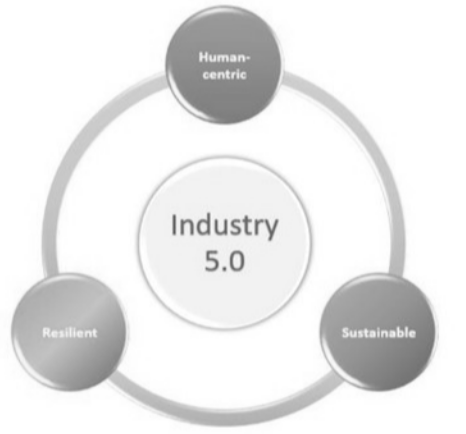
It is essential that the SME industry survives upheavals and provides assistance when needed. A sustainable approach can help SMEs recover, grow, and contribute to long-term survival.
Big data analytics makes it easier for businesses to manage large amounts of data. Businesses can use this emerging trend to manage complex datasets, democratize insights, and empower workers. Small companies are not using big data analytics because they are late adopters.
E.V. manufacturers can become more prosperous with a digital backbone. Contrary to established car companies, E.V.s are disrupting the market. The maturity model assessment must include electric vehicles (E.V.s) to measure the transition to zero-emission cars. Customers can use big data about charger networks to recharge their batteries en route. In electric vehicles, GPS navigation systems can incorporate charger information and directions to anticipate battery recharging needs.
In addition to 5G reducing data transmission latency, big data and IoT require fast data transfer rates, and factory automation relies heavily on real-time operations. Using big data can improve visibility, insights, and control for manufacturers. It can also improve production processes, reduce downtime, improve workplace safety, and predict maintenance issues. IoT and big data applications in industries like industrial automation get a fillip due to 6G networks. They will be able to deliver exceptionally high-performance connectivity. In 6G, machines and processes can exchange data instantly and with ultra-low latency. Augmented analytics will be a big trend in big data, with machine learning, A.I. helping, and advanced sensing allowing for low-latency, fast communications.
10. Future challenges and opportunities
There is constant refinement and development of new Big Data models, opening up new research opportunities; Big Data maturity models and the area of Big Data are constantly evolving. The Big Data domain will undoubtedly gain acceptance in the following years due to its potential for generating business value. Today, Big Data is still a relatively new domain, but it will undoubtedly prove to be a valuable area in the future. Big Data is becoming part of everyday business activities. Strategic Technological and critical business solutions will continue to evolve and improve. As Big Data evolves, new business approaches will emerge. As a result, future research should explore how Big Data contributes to business value creation. In addition, conducting a new benchmarking study for Big Data maturity models in the coming years would be worthwhile in assessing the new models and reevaluating the old models in light of evolutionary changes. Understanding the evolution of Big Data maturity modeling practices would be valuable.
Organizations operate, make decisions, and gain insights using big data, and as we look to the future, we see that it holds immense potential. With digital transformation, enterprises can improve their competitiveness. Big data technologies will increase The production of goods by 40% by 2035. Economic growth will average 1.7% across different industries. Intelligent manufacturing is complex for small and medium-sized businesses; they need not implement it better. Inventing must keep up, however. A study predicts that 75 billion IoT devices connect to the internet by 2025, and connecting all these devices to intelligence will be difficult. Organizations implement A.I. and IoT in production processes because of global competition. Intelligent manufacturing is the key to manufacturing’s ability to compete globally. New revenue streams will arise as big data, machine learning, and IoT interconnect [25]. Big data opens up a whole new era of possibilities in the future. Several concrete recommendations have already been provided for developing and implementing the big data maturity model to ensure successful future integration and advancements of big data within an organization.
11. Conclusions
This study aimed to devise a benchmarking process to assess the business value created by maturity models and good practices for maturity modeling. Therefore, business organizations could compare maturity models to identify the best model for evaluating and improving their Big Data maturity. The purpose of Big Data MMs has expanded to include evaluation of the implementation of Big Data. Before implementing Big Data, organizations must adopt mature designs to maximize their success. In addition, this paper provides essential insights that can help relevant stakeholders select more effective models for their organizations.
Plant floors are experiencing technologies that were once considered science fiction. Machine learning algorithms enable manufacturing plants to go beyond tracking operations and use Big Data for decision-making. By doing so, they can control inefficiencies, evaluate alternative strategies, and develop new protocols without simultaneously backing up the supply chain. Manufacturing will likely suffer significant consequences due to an uncertain and turbulent environment, such as mandatory closures, logistical bottlenecks, supply problems, and volatile consumption patterns. Embracing advanced digital technologies, like big data, is critical to manufacturers’ success. Scholars and practitioners are increasingly interested in big data due to the increasing amount of data collected and processed by firms. Digital transformation and big data are revolutionizing manufacturing, so organizations need a big data maturity model to evaluate their capabilities. SME maturity models need to be updated to meet the requirements of big data-focused SMEs. SME managers do not understand big data, like “unclarity regarding the benefits” and “insufficient understanding” of it.
- Salian, D.T., Sbeit, R., “Review of Digitalization Using Big Data Maturity Models: The Case of American Automotive SMEs,” 2023 IEEE 14th Annual Ubiquitous Computing, Electronics & Mobile Communication Conference (UEMCON), 2023.
- Cakir, A., Akın, Ö., Deniz, H. F., Yılmaz, A., “Enabling real time big data solutions for manufacturing at scale,” Journal of Big Data, 9(1):118, 2022, DOI: https://doi.org/10.1186/s40537-022-00672-6.
- “The big data bandwagon: How big data can benefit organizational practices,” Strategic Direction, 36(10):13-14, 2020, DOI: https://doi.org/10.1108/SD-08-2020-0144.
- Khan, Z., Vorley, T., “Big data text analytics: an enabler of knowledge management,” Journal of Knowledge Management, 21(1):18-34, 2017, DOI: 10.1108/JKM-06-2015-0238.
- Cappellesso, G., Thomé, K.M., “Technological innovation in food supply chains: systematic literature review,” British Food Journal, 121(10):2413-2428, 2019, DOI: 10.1108/BFJ-03-2019-0160.
- “So, are the geeks inheriting the earth?: Big data, big decisions and best practice for information management,” Strategic Direction, 29(9):12-15, 2013, DOI: https://doi.org/10.1108/SD-08-2013-0051.
- “The big data boost: Using proprietary big data to boost competitive advantage,” Strategic Direction, 37(5):20-21, 2021, DOI: https://doi.org/10.1108/SD-03-2021-0027.
- Rosangela de Fátima, P. M., Tereza Cristina Melo de, Brito Carvalho., “Examining the nexus between the vs of big data and the sustainable challenges in the textile industry,” Sustainability, 14(8):4638, 2022, DOI: https://doi.org/10.3390/su14084638.
- Dasari, S., Kaluri, R., “Big data analytics, processing models, taxonomy of tools, V’s, and challenges: State-of-art review and future implications,” Wireless Communications & Mobile Computing (Online), 2023, DOI: https://doi.org/10.1155/2023/3976302.
- “Maximizing the potential impact of competitive intelligence: Big data strategy lessons from Brazil’s private sector,” Strategic Direction, 36(9):5-7, 2020, DOI: https://doi.org/10.1108/SD-06-2020-0126.
- “The next big thing?: How big data is shaping firms’ international strategies,” Strategic Direction, 35(4):10-12, 2019, DOI: https://doi.org/10.1108/SD-01-2019-0004.
- Peinado-Asensi, I., Montés, N., García, E., “Industrial internet of things and big data techniques for the smart press shop 4.0 development in automotive industry,” IOP Conference Series. Materials Science and Engineering, 1284(1):012012, 2023, DOI: https://doi.org/10.1088/1757-899X/1284/1/012012.
- Llopis-Albert, C., Rubio, F., Valero, F., “Impact of digital transformation on the automotive industry,” Technological Forecasting and Social Change, 162, 2021, DOI: 10.1016/j.techfore.2020.120343.
- Alstyne, M.W.V., Parker, G.G., “Digital Transformation Changes How Companies Create Value,” Harvard Business Review, 2012.
- “Big data performance boost: Improving dynamic capabilities through big data analytics,” Strategic Direction, 39(7):7-8, 2023, DOI: https://doi.org/10.1108/SD-06-2023-0073.
- “The big data bandwagon: How big data can benefit organizational practices,” Strategic Direction, 36(10):13-14, 2020, DOI: https://doi.org/10.1108/SD-08-2020-0144.
- “The big data boost: Using proprietary big data to boost competitive advantage,” Strategic Direction, 37(5):20-21, 2021, DOI: https://doi.org/10.1108/SD-03-2021-0027.
- Qasim, A. N., Nasir, N., Jamshed, S., Naz, S., Ali, M., Shahzad, A., “Big data management and environmental performance: Role of big data decision-making capabilities and decision-making quality,” Journal of Enterprise Information Management, 34(4):1061-1096, 2021, DOI: https://doi.org/10.1108/JEIM-04-2020-0137.
- Hu, J., Gao, S., “Research and Application of Capability Maturity Model for Chinese Intelligent Manufacturing,” Procedia CIRP, 83:794-799, 2019, DOI: https://doi.org/10.1016/j.procir.2019.05.013.
- Bozic Yams, N., Richardson, V., Shubina, G., Albrecht, S., Gillblad, D., “Integrated A.I. and Innovation Management: The Beginning of a Beautiful Friendship,” Technology, 10:5-18, 2023, DOI: 10.22215/timreview/1399.
- Ansari, I., Barati, M., Sadeghi Moghadam, M. R., Ghobakhloo, M., “Readiness model for new technology exploitation,” The International Journal of Quality & Reliability Management, 40(10):2519-2538, DOI: https://doi.org/10.1108/IJQRM-11-2022-0331.
- Chen, W., Liu, C., Xing, F., Peng, G., Yang, X., “Establishment of a maturity model to assess the development of industrial A.I. in smart manufacturing,” Journal of Enterprise Information Management, 35(3):701-728, 2022, DOI: https://doi.org/10.1108/JEIM-10-2020-0397.
- Paschou, T., Rapaccini, M., Peters, C., Adrodegari, F., Saccani, N., “Developing a Maturity Model for Digital Servitization in Manufacturing Firms,” 2019.
- Valerio, P., “Industry 5.0 adds human-centric, sustainable, and resilient concepts to the industrial revolution,” IOT Times, 2021, URL: https://iot.eetimes.com/industry-5-0-adds-human-centric-sustainable-and-resilient-concepts-to-the-industrial revolution/.
- Friedenberg, M., “Our next great era: IDG enterprise’s CEO spells out the future of big data, mobility and the impact of 50 billion connected devices by 2020,” CIO, 26(11): 2013.
No. of Downloads Per Month